Natural Language Processing (NLP) has become a real game changer for businesses—some more than others. But no matter what your business or sphere of work, there are many ways in which NLP can help ease your workload, improve accuracy, and save money. Here are ten real-life use cases for NLP in business.
1.Document (Text) Classification
Text is everywhere: emails, chats, website content, social media posts, blog posts…there’s a lot of it out there and it’s mostly unstructured, making it hard to extract any kind of value. Which is where NLP comes in. Text classification or text categorization is simply the process of segregating text into specific groups. NLP automates this process and assigns pre-defined tags to each based on the content of each document. This makes the documents easier to manage, sort, and mine for specific information. For instance, say you receive a mountain of emails every day. It’s all mixed up and you can’t risk deleting it en masse for fear of throwing out the baby with the bathwater. NLP can classify these documents in a flash, by separating them into various categories, based on the occurrence and frequency of certain keywords. Text that has keywords pertaining to more than one category can simply be categorized under both classes.
2.Sentiment Analysis
Every time we use emojis to register our approval (or dislike) of certain posts on social media or post reviews of a product or service we are engaging in expressing our sentiments. This is invaluable for brand/business owners, who use it to inform their business/brand strategies. But sentiment analysis can go far beyond this. For instance, it can be used to determine market/stock trends, based on the frequency of certain keywords.
Sentiment analysis can also be used by HR departments—using posts, feedback surveys, reviews, etc.—to gauge employee satisfaction with their job roles and strategize accordingly to enhance attrition.
3.Data Mining
Every day we generate a massive volume of data, most of it unstructured, but no less valuable. To unearth this intelligence, however, it is necessary to sift through tomes of text—next to impossible to manage manually, but a breeze for NLP. NLP solutions can automate the extraction of specific data related to a particular topic from any source, be it text documents, databases, or websites.
NLP can be used to train models to classify documents according to such variables as ‘date’, ‘person’, geography’, organization, or an ‘event’. The Fintech industry, for instance, uses NLP models to extract data from statements, reports, claims, and forms. This reduces the chances of error associated with manual entry, increases accuracy, and automatically sorts documents according to custom rules.
4.Chatbots
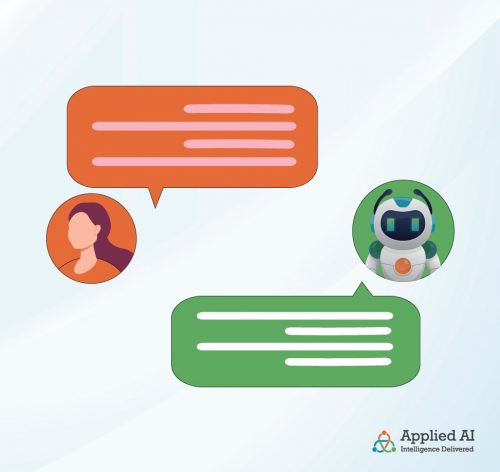
NLP can give chatbots conversations near human sentience
Who amongst us hasn’t thrown up their hands in exasperation after a frustrating session with a chat application? Conventional chatbots—even those personalized with names, and anthropomorphic features—are just not up to the task of conversing with humans. Enter NLP. Chatbots built using NLP solutions are now almost sentient. They can understand intent, converse in context and learn from previous conversations, making each interaction better than the one before. Bot models can be built and trained to perform specific conversations without needing to collect and tag extensive datasets for their training. The uses for chatbots, and voice assistants is seemingly limitless, from conducting interviews for HR to performing certain actions like Siri does. And since it is designed to take an automatic learning approach, it just gets better the more you use it.
5.Text Summarization
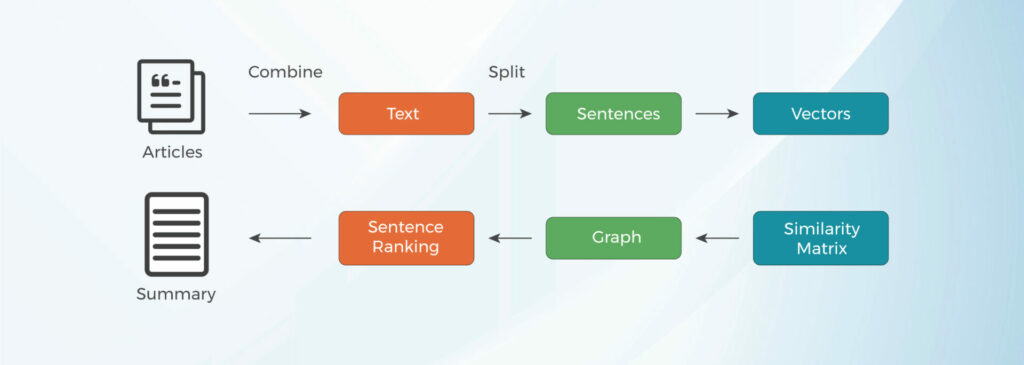
Typical process of automated text summarization
Information overload and Content Shock are very real. The continuous flood of information can be hard to process or use effectively. Summarization allows you to condense this voluminous information into convenient formats that are easy to digest. Summarization can be particularly helpful in the following cases:
- Media Monitoring
- RSS feeds
- Website content—with a view to SEO.
- Internal knowledge—typically stored as unstructured data
- Financial research—earning reports, financial news, et al.
- Contract analysis
- Repurposing long-form content for social media marketing
- Email—enabling us to skim emails quickly
Like to know how summarization can help you? Ask us in the comments below, or reach out to our NLP experts.
6.Custom search applications
Nobody (well, almost nobody) really knows for certain how Google’s algorithms might change in the future, but one thing is certain: NLP will play a big role in redefining how search engine marketing works. With NLP, search queries can better understand the intent behind the search…for instance homophones like accept and except; or homonyms like right (as in correct) and right, as in the opposite of left. The implication for SEO marketers is clear: content is doubly important now, as in content that is designed for human consumption rather than search bots.
For business organizations, search engines play an important role too. They help search emails, documents, etc. When searching for anything using any query, the search widget creates a list of documents matching the query. And it uses an index of documents/vocabulary to do this. Enterprise search engines that are built using NLP models will not face typical problems such as longer search queries or words that have dual meanings. A custom search engine is thus able to quickly and accurately find documents according to the searcher’s real intent.
7. Text similarity
Plagiarised content is a huge problem, not just in academia but in everyday writing as well—this article, for instance. Using NLP it is easy to build applications that flag documents that show a high degree of similarity—not merely by matching words, but by searching for semantical similarities, too.
8. Language translation
The idea of machine-translation has always been around; the challenge lay in the complexity inherent in any language. Then in the late 1990’s Alta Vista—a search engine that was later bought and merged into Yahoo—launched the world’s first web-based translation tool: Babel Fish. While its translations do present users with a gist of the translated material, it is far from perfect. It doesn’t play well with idioms and is often confounded by homonyms and other figures of speech. Thus language translation remained a dream until Google launched Google Translate. Google Translate, which originally used statistical machine translation, now uses neural machine translation—translating entire sentences at a time, rather than just piece by piece. This allows it to use a broader context to identify the most relevant translation, in effect, allowing it to speak almost like a human and in real-time.
NLP-powered language translation allows you to translate instantly translate content pieces, such as web pages, into selected languages with amazing accuracy.
9. Conversational AI
Conversational AI is the term describing the technology that is at the heart of virtual assistants like Cortana, Alexa, and Siri. These applications rely on NLP to understand the intent behind user queries and generate an accurate response. Such chatbots are immensely useful in answering customer queries, and even assisting in rule-based tasks like booking flights. Their accuracy and 24×7 availability spell lower costs, and higher user engagement. They can be used to excellent effect in reducing workloads on call centers, allowing customer service employees to focus on more complex issues.
- Automated Speech Recognition (ASR)
ASR works by converting human speech from analog acoustic sound waves to digital forms that can be read by machines. It splits speech into individual sounds—called tokens—analyzes each sound, and uses NLP + deep learning algorithms to identify the best-fit word in the selected language, before converting the sound into text. Many Smartphones today offer speech recognition applications (Siri, for example) to enhance accessibility beyond texting.