The term ‘moonshot’, in technology, refers to an ambitious, groundbreaking project; one undertaken without assurance of short-term profitability, but which, if it works out, can change the world in a definitive way. The term probably owes its origin to the idiom “shooting for the moon”— implying an outside chance of success. AI has always held out the potential for moonshots. And in 2013, the MD Anderson Cancer Center launched its own moon shot: a project that aimed to diagnose certain forms of cancer using IBM’s Watson cognitive system, and recommend treatment. In 2017, after exceeding costs of US$62, the project was put on hold. While this is a sadly familiar ending with most moonshots, the technology itself had a lot of other, less ambitious, uses to offer. In contrast with the long shot of moonshots, there are several lower hanging fruit that AI/Ml technologies offer, and one that has seen the greatest use in recent times is a subsection of AI called: Natural Language Processing (NLP). And the fintech industry has been quick to see its benefits and put it to use.
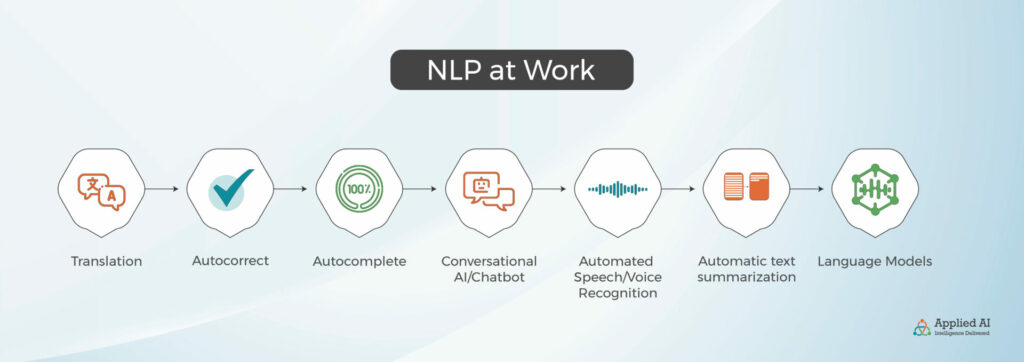
NLP use-cases In fintech
Credit scoring
One of the most basic uses of NLP is credit scoring. This is a statistical analysis that lenders, banks, and insurers routinely conduct, prior to sanctioning a loan, to ascertain the creditworthiness of an individual or a business. The process was, traditionally, a manual one involving poring through multiple documents, such as income, investments, expenses, etc., and extracting data that can be used to analyze creditworthiness.
NLP streamlines, simplifies, and speeds up the process by extracting relevant data from unstructured documents and feeding it to credit scoring software to determine the credit score. That’s not all. NLP can also be used to extract supplementary information from personal profiles , such as social media accounts, mobile applications, etc.
Managing insurance claims
NLP can be used in combination with Optical Character Recognition (OCR) software to analyze insurance claims. This approach can be used to comb through structured and unstructured text data in order to detect the right information to process insurance claims. The application then feeds the information to an ML algorithm, which labels the data according to the specific sections of the claim application form, with the terminology that is commonly filled into it.
Financial auditing
Financial auditing processes can be simplified and speeded up using NLP to automate certain parts, for instance:
Screening of financial documents
Classifying financial statement content
Identifying similarities and differences in documents
This, also enables flagging of anomalies in financial statements.
Fraud detection
NLP plus ML and predictive analytics can be easily used to detect fraud and misinterpreted information from unstructured financial documents.
In a study, researchers successfully used NLP linguistic models to identify deceptive emails—identified by a certain frequency or reduced frequency of first-person pronouns and specific words, words connoting negative emotions, and action verbs. Using a classifier algorithm, researchers were able to analyze linguistic features of annual reports, including voice, active versus passive tone, and readability, and detect any association between these features and fraudulent financial statements.
Loan & mortgage fraud detection
Loan and mortgage applications see a lot of criminal activity, such as the use of a stolen or fake identity to apply for a loan. Fraudsters establish credit history by using the identity to set up a number of credit lines, and finally max out all the credit lines and disappear. Such applications take a long time to detect and even longer to deny. Using a logistical regression model—easily explainable to regulators—with features like loan transaction details, bank statements, credit score, history on banking activities and credit history, bank account details, borrower’s identity, age, income and asset information, and other public information, the AI-powered model was able to flag fraudulent applications as good or greater accuracy than humans
AI-powered NLP technology can also be used to make data-driven decisions that give businesses a competitive edge. By searching real-time private data stored on platforms like Evernote, OneDrive, and SharePoint, businesses can acquire new customers.
Another business area where NLP solutions can make a huge difference is customer service. One UK high street bank saw this in action when they integrated an AI-powered automatic email responder. The solutions automated common queries, sending them to the right department without manual intervention. This enabled the bank to speed up replies with 95% accuracy. And with more and more training data generated with each interaction the scope for fine-tuning the model is infinite.
Email assistants are also able to conduct sentiment analysis on data, using keywords, phrases, and combinations to identify such aspects as emotion and tone of voice. These findings are helping businesses modify their own interactions, resulting in a much higher level of customer satisfaction
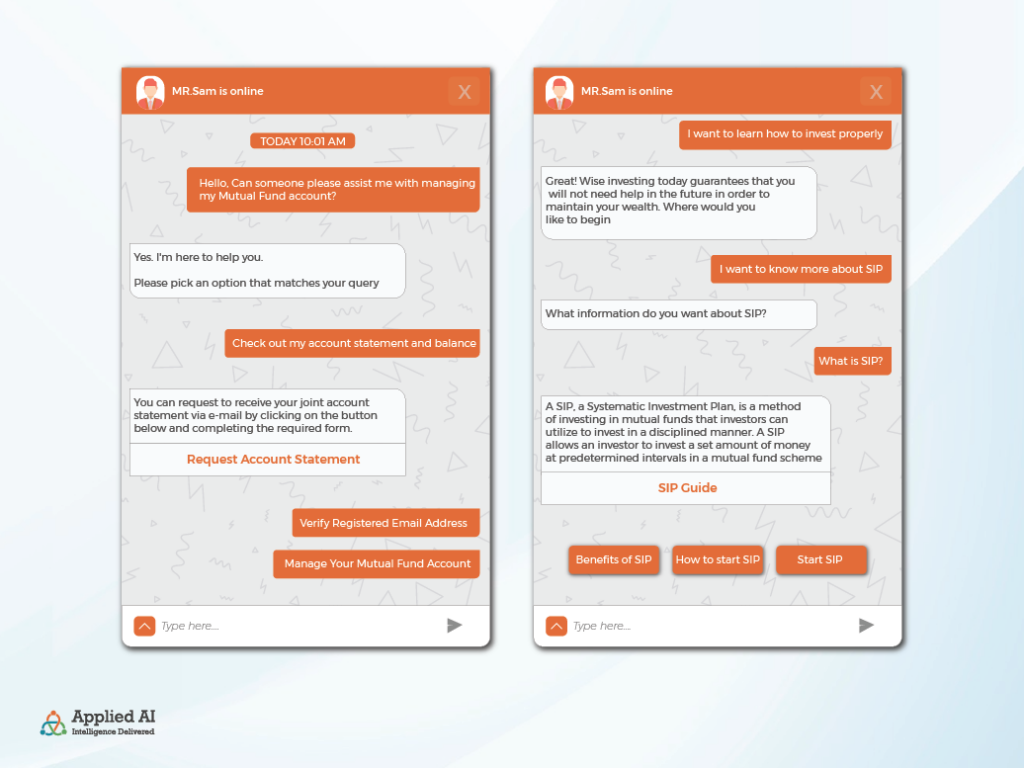
Voice assistants and chatbots
AI/ML-powered chatbots and voice assistants can deliver near-sentient experiences, easing the pressure on human staff, while improving customer experiences.
Conclusion
In addition to the above use cases, there are many, many other areas where AI/ML technologies like NLP can raise competitiveness, improve bottom lines and enhance experiences, not just for business stakeholders and their customers but also for their staff.
NLP in particular can be leveraged on interview platforms to analyze candidate sentiment, screen uploaded documentations, check for references, detect specific keywords that reflect positive or negative behavior, and more. NLP is also particularly useful in such areas as detecting employee sentiment, for e.g. detect job satisfaction, racial/sexual bias, etc. from feedback surveys, social media posts and comments, etc. this can help HR pre-emptively address conflict areas, build trainings, engage employees, and create the optimal work culture.