-
- Initial – Ad hoc, reactive use of technology
- Repeatable – Some processes, basic KPIs emerging
- Defined – Standardized, documented, metrics-driven
- Managed – Data-led performance tuning
- Optimized – Scalable innovation, continuous improvement
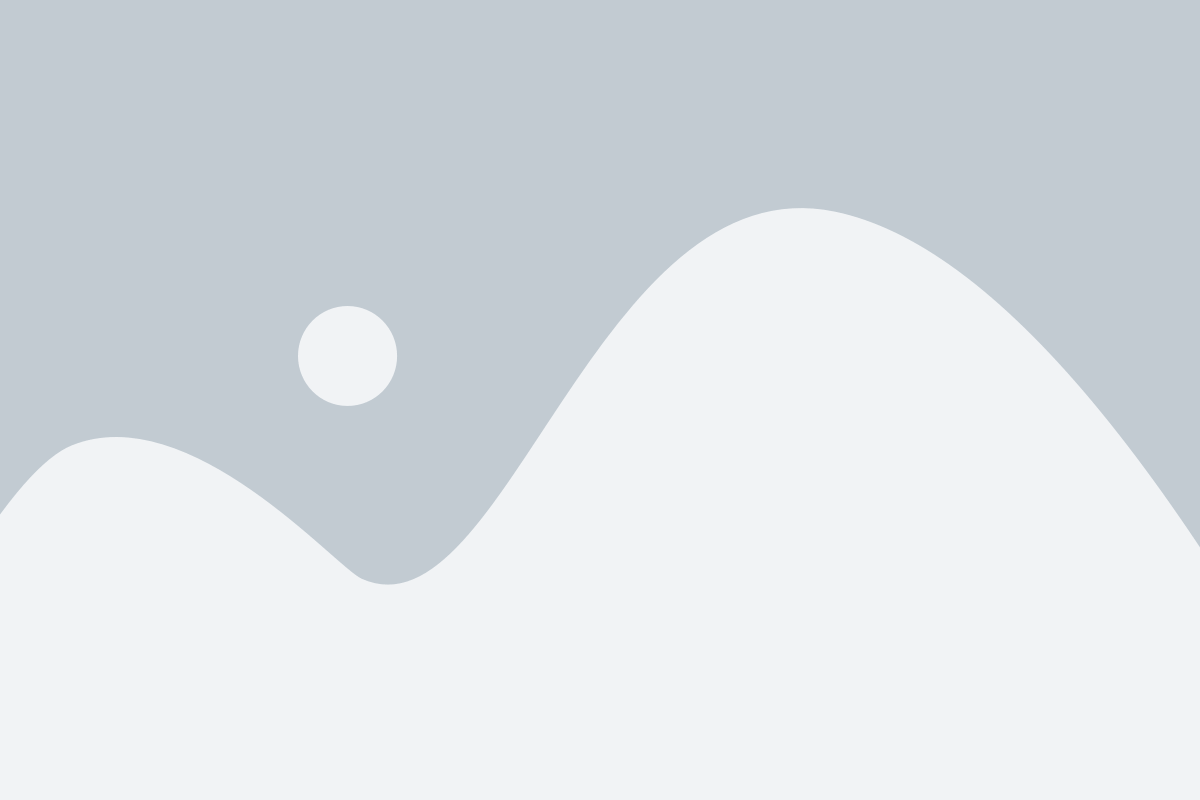
The AI Maturity Journey
Level 1: Awareness
Level 2: Active
Early adopters begin experimenting—often through small-scale pilots or proof-of-concepts. Data scientists might explore models using Jupyter notebooks or public ML libraries. Success is sporadic, and infrastructure is limited.
Level 3: Operational
AI is embedded into specific business processes. There’s usually a dedicated team of ML engineers. Models are trained, deployed, and monitored. Infrastructure like MLOps, versioned data pipelines, and reproducibility frameworks become essential.
Level 4: Systemic
AI starts to reshape the way business operates. Teams no longer view it as a tool for “efficiency” but as a catalyst for business model innovation. Think recommendation engines, dynamic pricing, or automated underwriting.
Level 5: Transformational
How to Start: Making AI Adoption Practical
- What decisions drive our business?
- What data do we collect (or can we collect)?
- What value will automation unlock?
1. Explore Current Practices
Freeing human teams from routine decisions unlocks bandwidth for creativity, empathy, and innovation.
2. Envision New Decisions
Five well known AI Maturity models
1. MIT Sloan's Four-Stage AI Maturity Model
- Stage 1: Experiment and Prepare
Organizations focus on educating their workforce, formulating AI policies, and experimenting with AI technologies to become more comfortable with automated decision-making - Stage 2: Build Pilots and Capabilities
Companies develop AI pilots that create value for both the enterprise and its workers, focusing on building capabilities and infrastructure - Stage 3: Scale and Transform
AI initiatives are scaled across the organization, transforming business processes and creating new value propositions. - Stage 4: Innovate and Lead
Organizations leverage AI to drive innovation, leading their industries with AI-powered products and services.
2. Accenture's AI Maturity Framework
3. Boston Consulting Group's AI Maturity Matrix
4. ServiceNow & Oxford Economics' Enterprise AI Maturity Index
This index, based on a survey of 4,470 executives, measures AI maturity across five key pillars: strategy, data, technology, talent, and governance. It offers a comprehensive view of how organizations are adopting AI, the challenges they face, and the best practices that lead to successful AI integration.
5. AIM Research & Hansa Cequity's Generative AI Maturity Framework
Case Study: AI for Smarter Sales Teams
The result?
- Better targeting
- Higher conversion
- More productive reps
AI Use Cases That Deliver
- Recommendation: Offer the next-best action, product, or service
- Anomaly Detection: Spot outliers in performance, security, or user behavior
- Segmentation: Tailor engagement based on user behavior, intent, or profile
- Forecasting: Predict outcomes, trends, and potential risks
Recommended Model: MIT Sloan’s Four-Stage AI Maturity Model (Enhanced for Decision Intelligence)
Applied AI's AI Maturity Model – Focused on Decision Intelligence
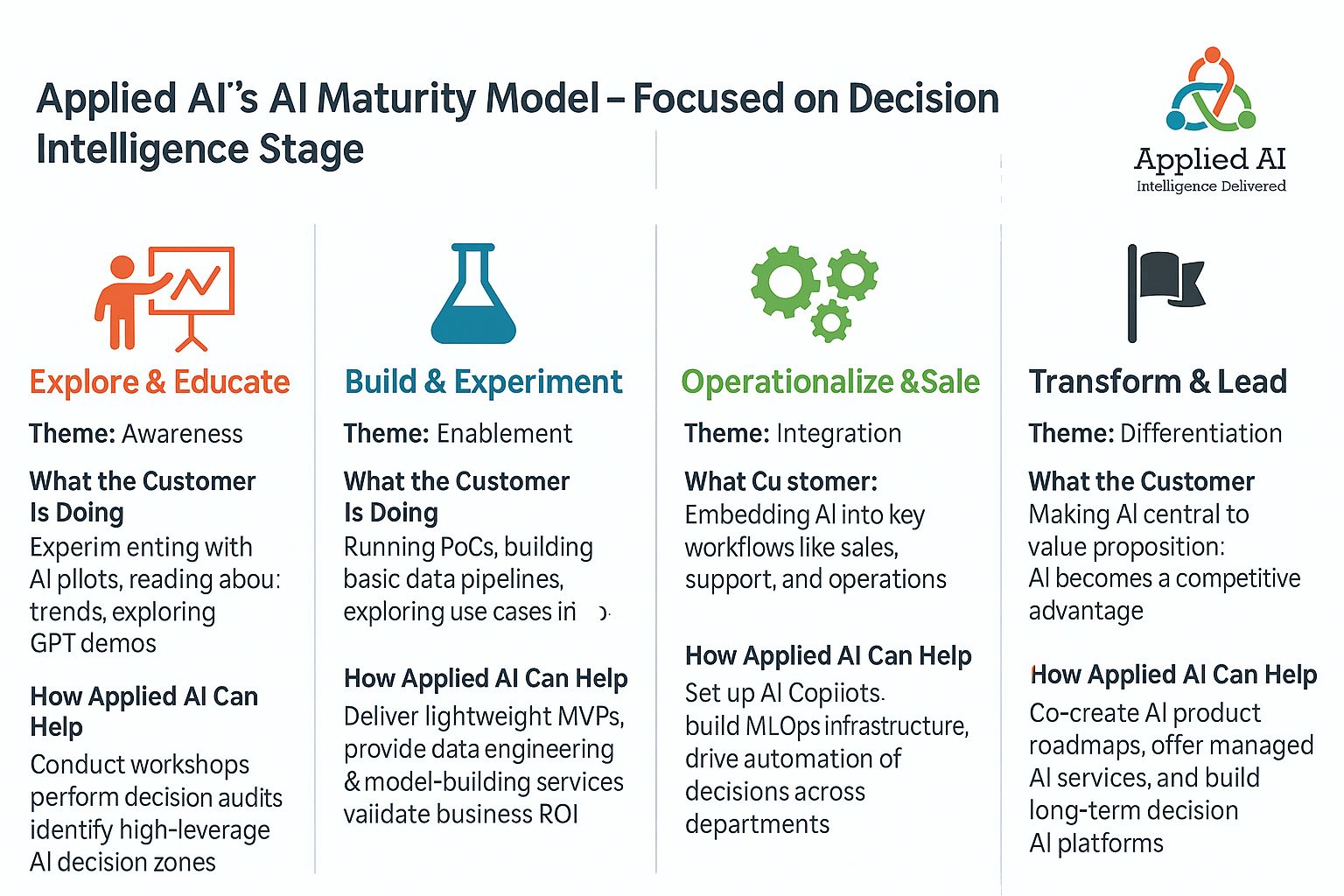
Stage | Theme | What the Customer Is Doing | How Applied AI Can Help |
---|---|---|---|
1. Explore & Educate | Awareness | Experimenting with AI pilots, reading about trends, exploring GPT demos | Conduct workshops, perform decision audits, identify high-leverage AI decision zones |
2. Build & Experiment | Enablement | Running PoCs, building basic data pipelines, exploring use cases in silos | Deliver lightweight MVPs, provide data engineering & model-building services, validate business ROI |
3. Operationalize & Scale | Integration | Embedding AI into key workflows like sales, support, and operations | Set up AI Copilots, build MLOps infrastructure, drive automation of decisions across departments |
4. Transform & Lead | Differentiation | Making AI central to value proposition; AI becomes a competitive advantage | Co-create AI product roadmaps, offer managed AI services, and build long-term decision AI platforms |
Why This Works for Strategic Partnerships with our clients?
- Strategic Framing: Starts with vision and decision-making, not just model accuracy or tech
- Flexibility: Tailored to each customer’s current maturity
- Value-Based: Positions Applied AI as a business-value enabler, not a software vendor
- Scalability: Sets up long-term engagement: PoC → MVP → Integration → Co-innovation
Final Thought: The Shift to Information-Driven Strategy
AI isn’t a magic bullet. It’s a tool—and like any tool, its value depends on how thoughtfully it’s applied.
At Applied AI Consulting, we help businesses move beyond the hype to build sustainable AI practices that transform operations, enhance decision-making, and drive real value. Whether you’re at Level 1 or Level 4, the path forward is clear:
Think in terms of decisions, not models. Think in terms of value, not vanity.
If you’re ready to explore your AI maturity, let’s talk.
WhatsApp: +91 9923417213
sanju@appliedaiconsulting.com OR vijay@appliedaiconsulting.com